In 1769 the Austro-Hungarian Baron Wolfgang Von Kempelen arrived at the Schonbrunn Palace in Austria in order to impress the empress Maria Theresa. For this purpose, he built a machine in the image of a Turkish man, complete with a mustache and a turban, and told the empress that this doll could beat any man at chess – and so it was.
For more than 80 years, the “automatic Turk” defeated various chess players, among them Napoleon and Benjamin Franklin. In 1854, the machine caught fire, and the son of its last owner revealed the Turk’s best-kept secret: there was always a short man, a world class chess expert, inside the machine.
Although the “Turk” turned out to be a fraud, it beautifully exemplifies how humanity has always been enchanted by smart machines. The idea that language and thinking can be converted into logical and computational actions, and through them the function of the human brain can be simulated, has its origins in a past even farther back than the automatic Turk – the ideas of philosophers such as Gottfried Wilhelm Leibniz in the 16th century. The ideas and the technology have come a long way since.
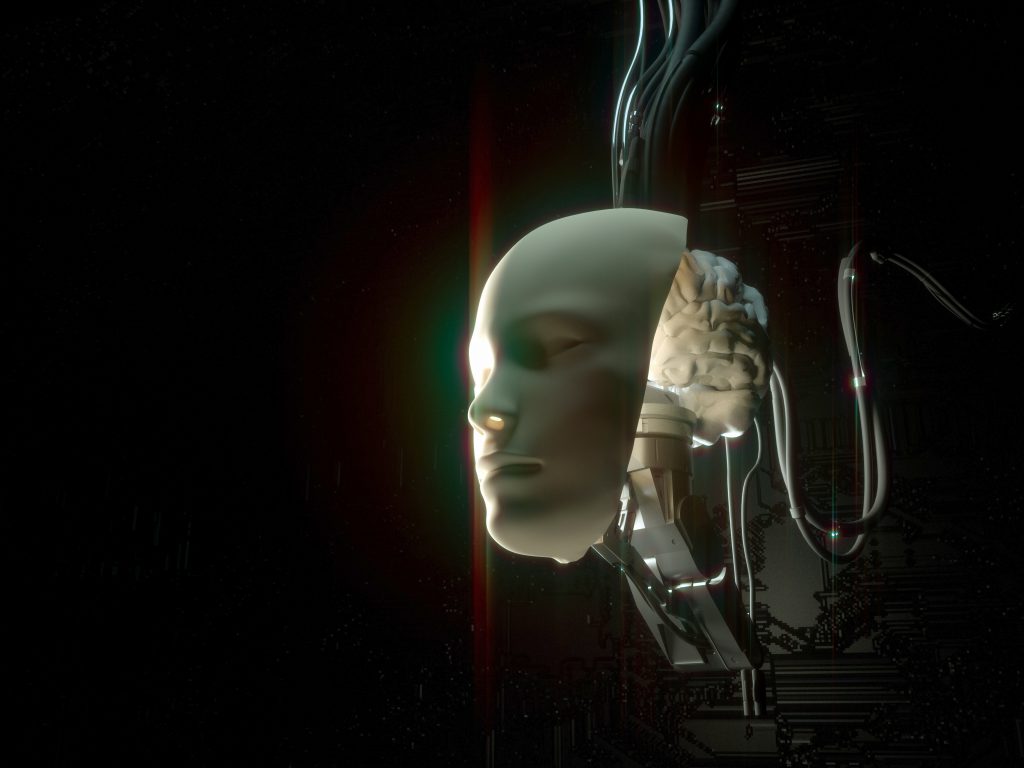
In the 1940s, Alan Turing, one of the forefathers of modern computing, succeeded in creating a fairly primitive chess program. But his preoccupation with the field attracted many researchers, who, in the late 1950s and early 1960s, laid the foundation for the world of artificial intelligence.
Within this supernatural title, artificial intelligence, or AI, there are many fields and sub-genres, all aiming for the same goal – to make the computer operate intelligently. Most are dependent on humans, which feed the computer new information. The classic example is Deep Blue, IBM’s famous computer, which in 1997 beat the then world chess champion Gary Kasparov.
“If we enter into the ‘brain’ of Deep Blue, we can see that it was essentially a chess calculator – one that had been fed a lot of information, and could consider around 200 million chess situations in a second,” explains Dr. Eli David, founding partner of the company Deep Instinct, and lecturer at Bar Ilan University’s computer sciences department.
“So it should not be taken lightly, it was indeed a tremendous success, but it was precisely at that time that many researches understood that some component of self-teaching should be incorporated into the field.”
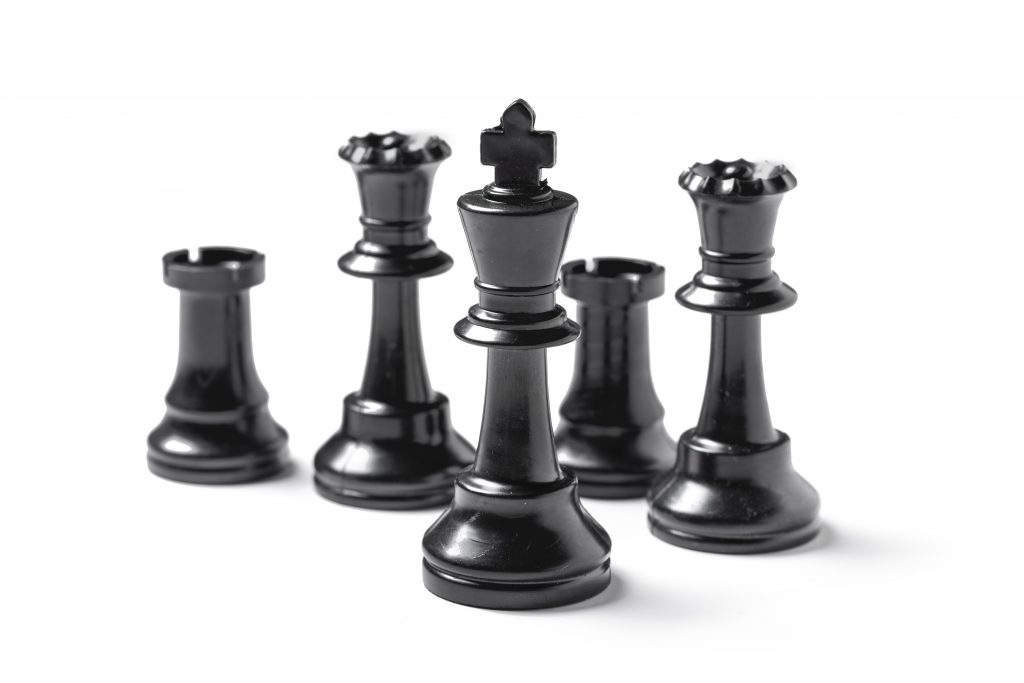
This is where the hottest field in today’s artificial intelligence arena comes in – machine learning. As opposed to other areas, here the computer doesn’t need humans to constantly feed it data to compute – it is able to learn more independently.
Different learning methods exist – the leading method until a few years ago was based on statistics. There is also the decision tree approach. But the big revolution in the world of artificial intelligence happened with the breakthrough in the method which took inspiration from the structure of the human brain – the “deep learning” or “neural network” method. This learning occurs in a way that mimics our brain function, through synapses (connections between nerve cells).
Hinton’s Revolution
Despite the attention it currently enjoys, the “deep learning” method is nothing new. It began in the 1970s. But it was very quickly marginalized, primarily because it did not provide good results, as opposed to other learning methods. “Nobody wanted to touch this field,” says Dr. David. Nobody except for a few individuals, including Professor Geoffrey Hinton, who was considered the most important researcher in the field of “deep learning”. For 30 years, he investigated the topic until the field’s biggest breakthrough several years ago which made it possible to train deep networks of 20-30 layers of artificial nerves instead of single layers. “The methods that we developed in the 1980s have long since gone beyond our wildest dreams,” says Hinton, who continues to do research in the field at the University of Toronto, Canada.
“The deep networks enable the machine to develop and learn a deep hierarchy of characteristics,” Dr. David simplified. For example, if a machine looks at a picture of an elephant, the lower levels of the nerve network identify basic characteristics, such as differentiating between colors, lines, textures, etc., and the higher levels connect these insights to more complex concepts, until the output layer turns up the name of the animal. In ‘shallow’ networks, there is not enough depth in order to learn complex characteristics.
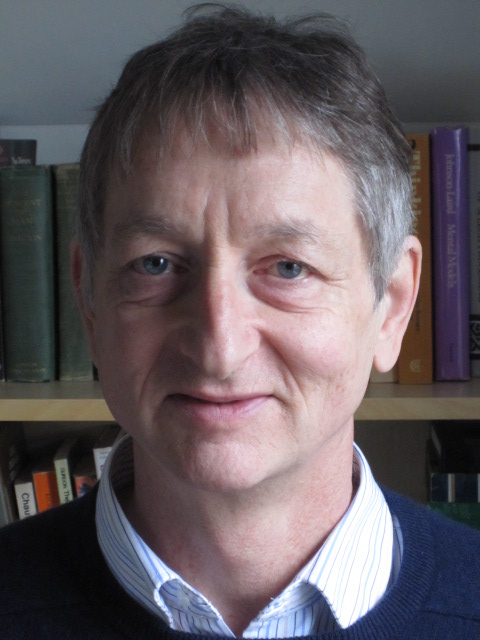
Alongside the breakthrough, with rare luck or coincidence, researchers discovered that strong graphics cards, the same cards that we insert into computers for computer games – those that are meant to quickly display millions of pixels on the screen – are brilliantly suited to the rapid calculation of values of millions of synapses. This discovery made the “training” of machines much more efficient than ever before. This market is aggressively controlled by Nvidia, which started out in the early 90s as a graphics card manufacturer for PCs and today supplies a growing number of chips to all of the technology giants competing in the AI arena – from Google, to Microsoft and Facebook to Amazon.
All of this brought about major momentum in all things pertaining to the AI industry. And if a few years ago, yearly improvement of a half-percentage was considered a tremendous achievement, now we are seeing improvements of ten and more percent in a year. Accordingly, thousands of companies that deal with the subject have popped up all over the world and are implementing these technologies in areas such as autonomous vehicles, telemedicine, digital marketing, financial and cyber services.
Intelligence Everywhere
“We believe that thanks to artificial intelligence, every field and each product is going to go through a change,” says Ami Luttwak, CTO of Microsoft Israel’s research and development center. “As evidence, the company has undergone significant organizational change in recent months, at the end of which two out of the company’s three development divisions are highly focused on AI. The company is betting on AI because we believe that it will become the basic infrastructure for everything we do and build.
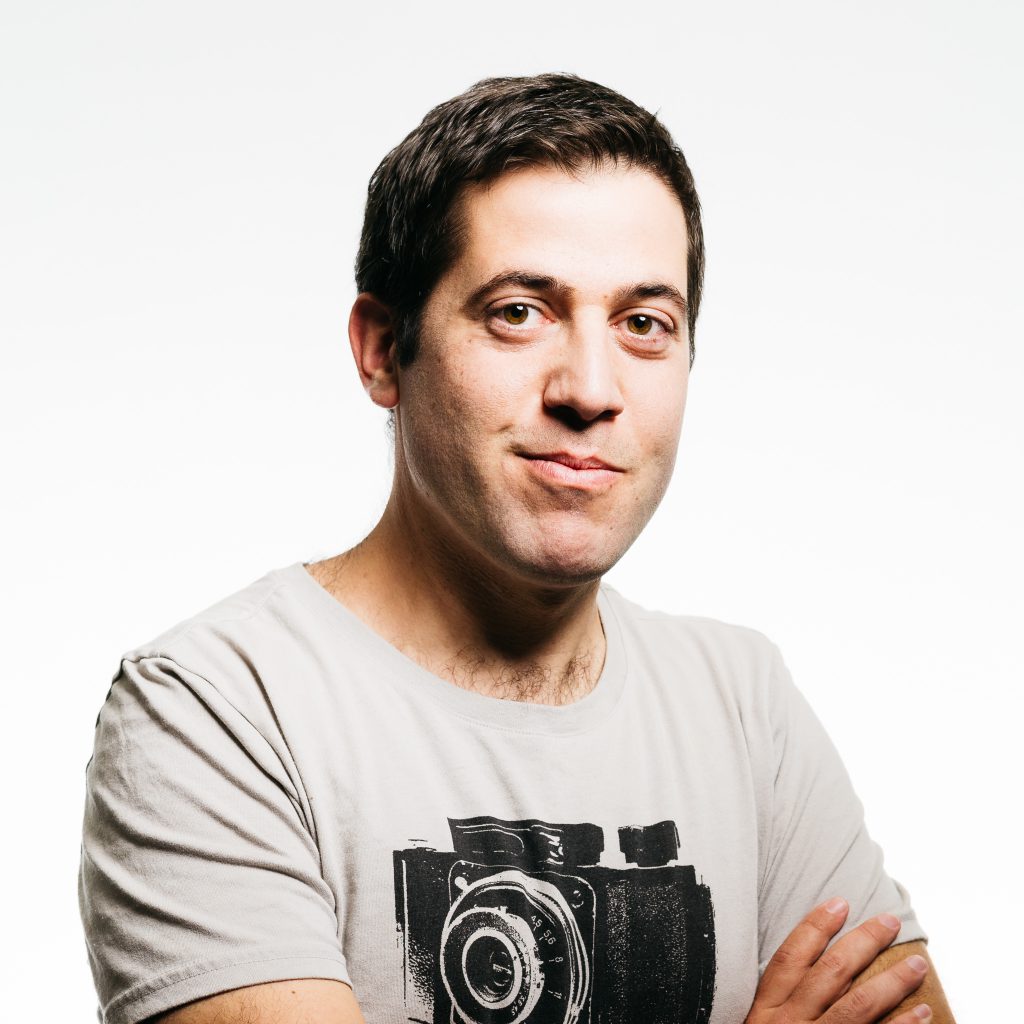
In the coming years, we will all learn to expect every device, every application, and every company we work with to be smart. Whether it’s a smart car, a smart refrigerator, or a chatbot from our bank, we will expect everything to understand us and act as automatically as possible.”
In fact, Luttwak says, “most of us already rely on technologies that use AI every day, without even knowing it. If you designed a PowerPoint presentation, it is definitely possible that you used AI. If you wrote a text on your phone, it’s safe to assume that you used AI.”
Microsoft Israel’s development center was established in the early 1990s. It is one of Microsoft’s three leading development centers set up outside of the USA, whose purpose is to serve as a development and innovation center for Microsoft which leverages the local talent and ecosystem. Luttwak (35) joined Microsoft in 2015, after it acquired Adallom for $320 million, a cloud information security company, that he established along with his two partners: Assaf Rappaport (who currently serves as CEO for Microsoft Israel’s development center), and Roy Reznik (ranked in Forbes 30 Under 30 in 2016).
According to Luttwak, a significant part of Microsoft’s AI activity is currently taking place in Israel. “There are many areas in which the development center in Israel is at the forefront of Microsoft’s AI development. There are advanced developments in the fields of education, CRM, security and healthcare and, of course, Cortana, the smart assistant whose great wisdom was developed in Israel.”
If you try to identify the direction that Microsoft is dealing with in AI, it is an attempt to “democratize” the technology. According to Luttwak, this has to do with one of the biggest challenges facing the industry – the lack of sufficient manpower. “There aren’t enough developers who know how to build advanced AI systems, and part of the solution is to make the AI services more accessible to all developers. Microsoft invests in AI services, which enable any developer to build smart applications, which can understand language, recognize faces, as well as talking bots, all without having to be an AI expert.”
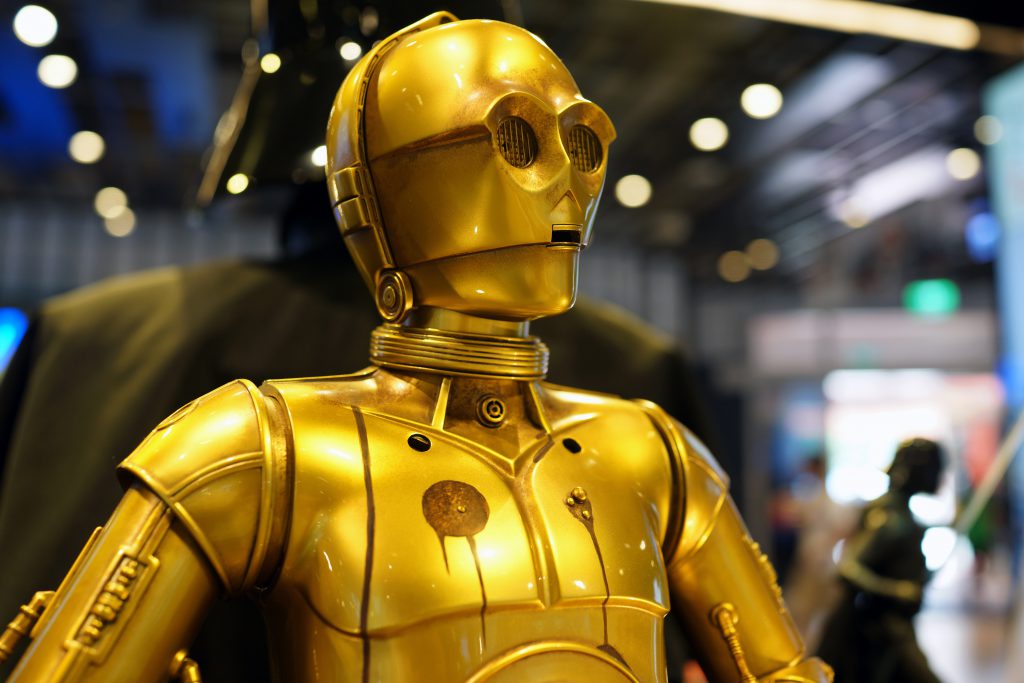
The Big Data revolution
“The idea that a machine can teach itself through data without a human programming it for what to focus on, was once considered crazy. Today it’s taken for granted,” says Professor Hinton, when he discusses the difference between “deep learning” and other learning methods in the field of “machine learning.”
In fact, the difference lies in the fact that other methods have an intermediate phase called the “characteristic extraction phase”. At this stage, an expert will remove all the important components for identification and prepare a list of characteristics which he or she inserts into the machine’s learning module. That is how a machine knows that in order to recognize a face, it must focus on, for example, the distance between the eyes.
In “deep learning” there is no intermediate phase. What is there instead? A hierarchical system of dozens of layers, which receives raw material, such as millions of pixels of a picture, and knows how to independently teach itself what to examine.
This kind of method requires a lot of information. Enter another revolution which has been underway in recent years and has enabled the machine learning method to flourish – the Big Data revolution, which came with the rise of the internet and particularly with the entrance of smartphones into our lives.
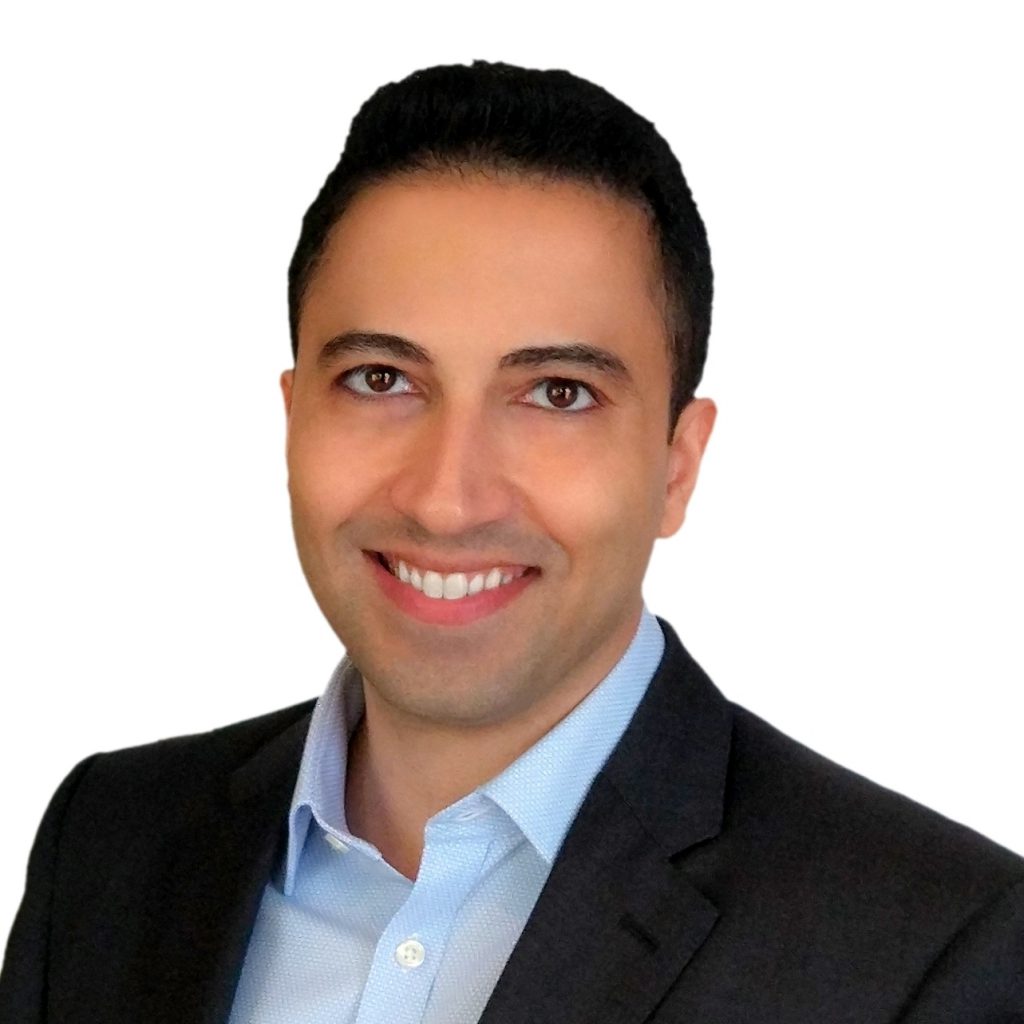
Actually, the fact that every one of us is walking around with a camera in their pocket, and uploading dozens if not hundreds of photos to social networks, has turned the world into a kind of machine learning paradise. And when you add to this the growing innovative technology and the cloud services which have enhanced data storage capabilities, we get the world of artificial intelligence that we have today. This connection also explains why the tech giants that control information are also the major leaders in developing AI technologies.
“Twenty years ago I wouldn’t have been able to operate my system,” says Dr. Kira Radinsky, visiting professor at the Technion and data science director for the company eBay (ranked in Forbes 30 Under 30 in 2016). “The algorithm already existed but the computing power was problematic and there wasn’t enough data. Today, for example, I can have a server farm of 1,000 processors in the cloud instead of maintaining a physical lab.”
Today, Radinsky, who has already sold a startup that she founded for tens of millions of dollars, is working on a new startup that uses artificial intelligence in the medical world. It will make it possible, for instance, to examine if the medicines approved for treatment of certain illnesses may also be effective in the treatment of other diseases. Moreover, AI can identify molecules with the potential to be the base for creating new drugs.
According to Radinsky, the greater the amount of information that the system is opened up to, the more it will improve – but the medical information of many clients is still inaccessible – which is an obstacle in the project’s development. “It’s not just a matter of regulators, but rather of organizations that do not want to give up that information,” says Radinsky. “A change in perspective regarding data will help us take the world another step forward.”
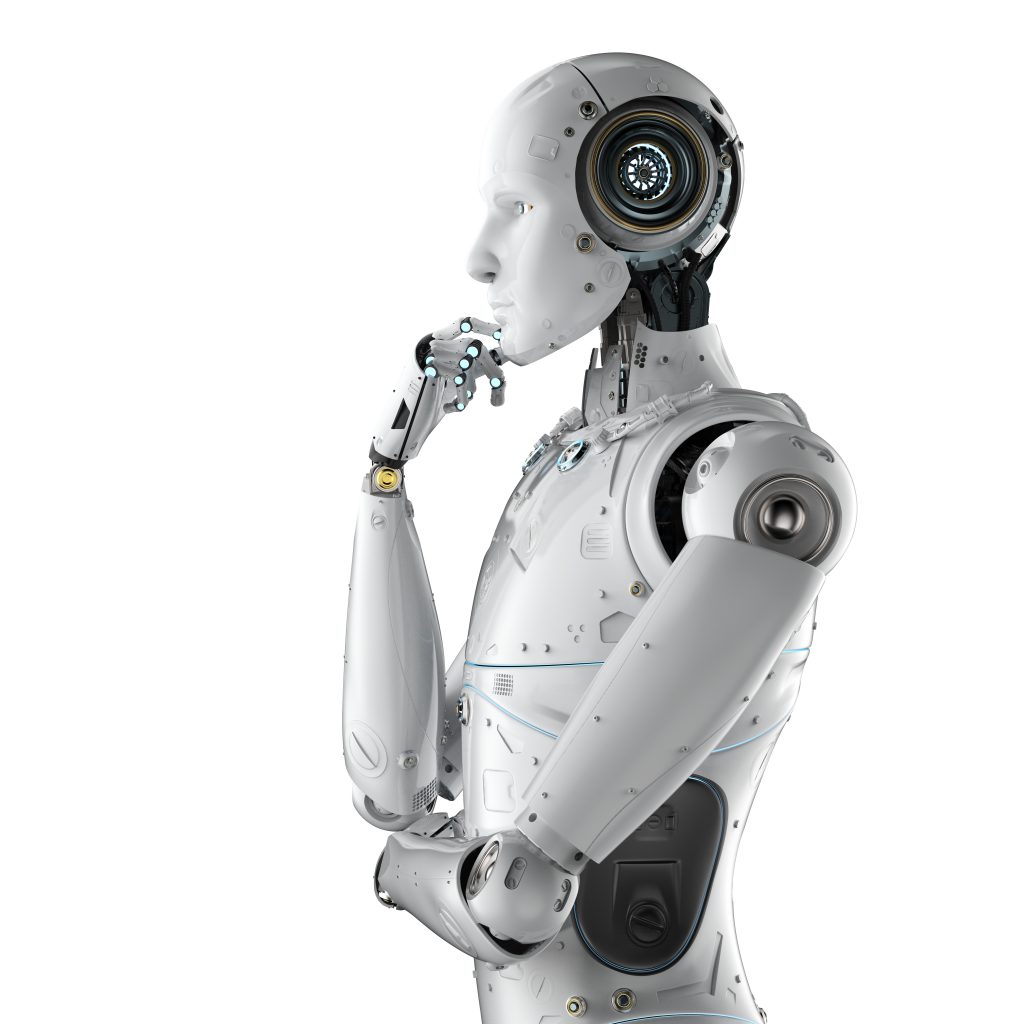
This problem, of access to large amounts of information, resonates for anyone currently engaged in developing AI technology. “Today’s technology requires that the learning system be fed with a huge amount of information – and more specific information, information about people, their identifiers and behaviors,” explains Luttwak. “This could create a significant obstacle for small companies or researchers who do not have the resources of big companies.”
Is there a Limit to Knowledge
Another problem with which researchers in the field must engage, is a methodological problem, in which it is hard to know what a self-teaching machine knows, which makes it hard to understand how it learned what it learned, which creates a problem for monitoring of acquired knowledge. According to Luttwak, in the coming years, AI abilities will be built into the systems that control our lives, whether they are systems for getting a job or medical care – and may contain errors, which could have far-reaching consequences. “Regulation must and is obliged to develop alongside the field. It won’t hold back development, but rather the opposite, will prevent future malfunctions,” he clarified. “To speed up public debate in the field, Microsoft published its approach in the book “The Future Computed”, in which it describes the future in 2038 and what might happen if ethics laws are not established today. For example, it offers a scenario in which future software companies only hire men because AI systems have learned that most developers are men and thus continue to maintain the status quo.
“The more sophisticated AI systems get and the larger a role they come to play in our lives, the more important it is that companies formulate and adopt clear principles to guide the people who build, use and implement AI systems.”
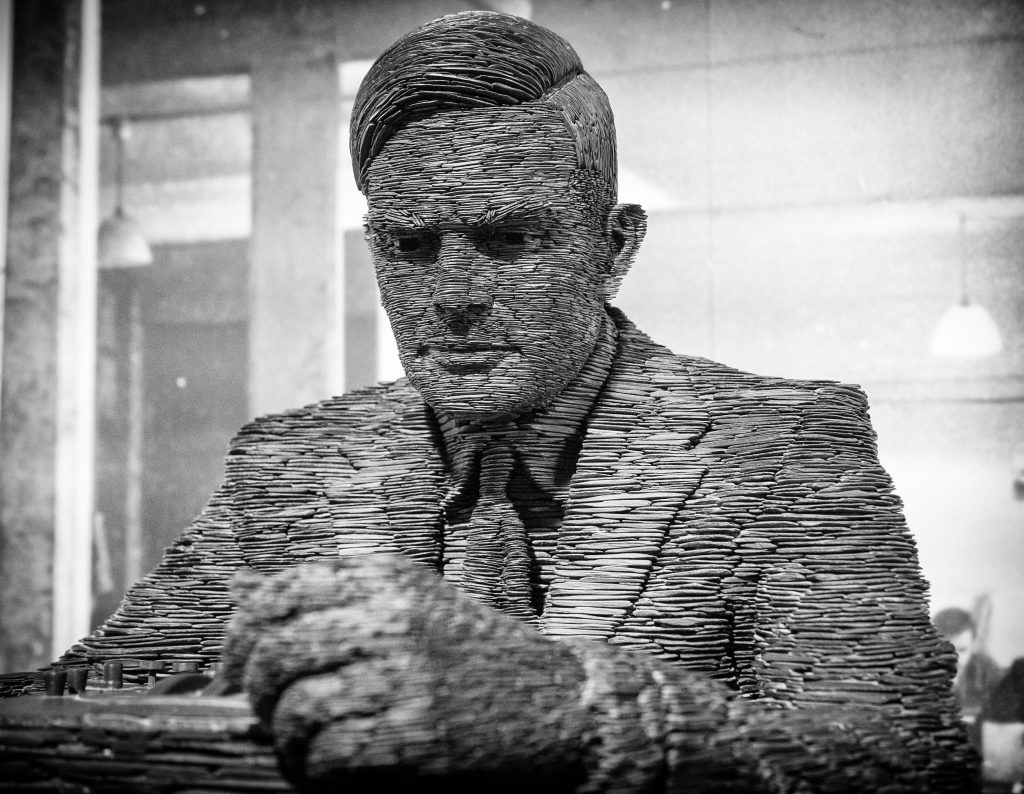
Distant Dream
Despite the ideal conditions that have bloomed in recent years, the real goals of today’s researchers are AI systems that can perfectly perform specific tasks (or as closely as possible to the human brain), such as photo recognition, talking, or even operations that require creativity such as writing songs. Nobody today is truly talking about a complete artificial brain, one that resembles a human, that can manage completely independently, like androids in sci-fi films.
Such a goal demands abilities that are still very far away. “While there have been enormous advances in recent years, we are still at a stage in which the computer must learn how to solve each specific problem one at a time,” Luttwak clarifies. “It does not yet have the ability to really understand what is happening, to connect between cause and effect and to intelligently make decisions. I believe that we will get closer to that in our lifetime, but it’s not as though it’s right around the corner.”
Prof. Shai Shalev-Shwartz, CTO of Mobileye and machine learning expert at the Hebrew University, explains that the difference between the human and artificial brain “lies in the fact that the human brain requires less information in order to learn. Moreover, the human brain can learn from information that is considered to be of lesser “quality”, while the best algorithms today require a great deal of high-quality information, that is, with little ‘noise’.”
“On the other hand, we see both in human brains and artificial brains, that as the brain grows quantitatively, the cognitive abilities grow,” adds Dr. David. “So maybe we aren’t there yet, but the scenario that artificial intelligence will reach and surpass our cognitive abilities is most likely.”
“It will be a long time until we get to the point where a machine looks, thinks and talks just like a human,” says Radinsky. And as for the fear that one day someone will create a machine that would hurt people? She suggests sticking to the words of researcher and innovator Dr. Andrew Ng: “to worry that a machine would hurt people is like worrying that there will be a population explosion on Mars, while humanity is just trying to get there.”
Translation by Zoe Jordan